Robust AMD Stage Grading with Exclusively OCTA Modality Leveraging 3D Volume
Published in International Conference on Computer Vision (ICCV) Workshop, 2023
In this paper, we experimentally verified that the OCTA projections, which ophthalmologists usually use for diagnosis, are easily affected by layer segmentation errors. Those errors degrade the classification performance.
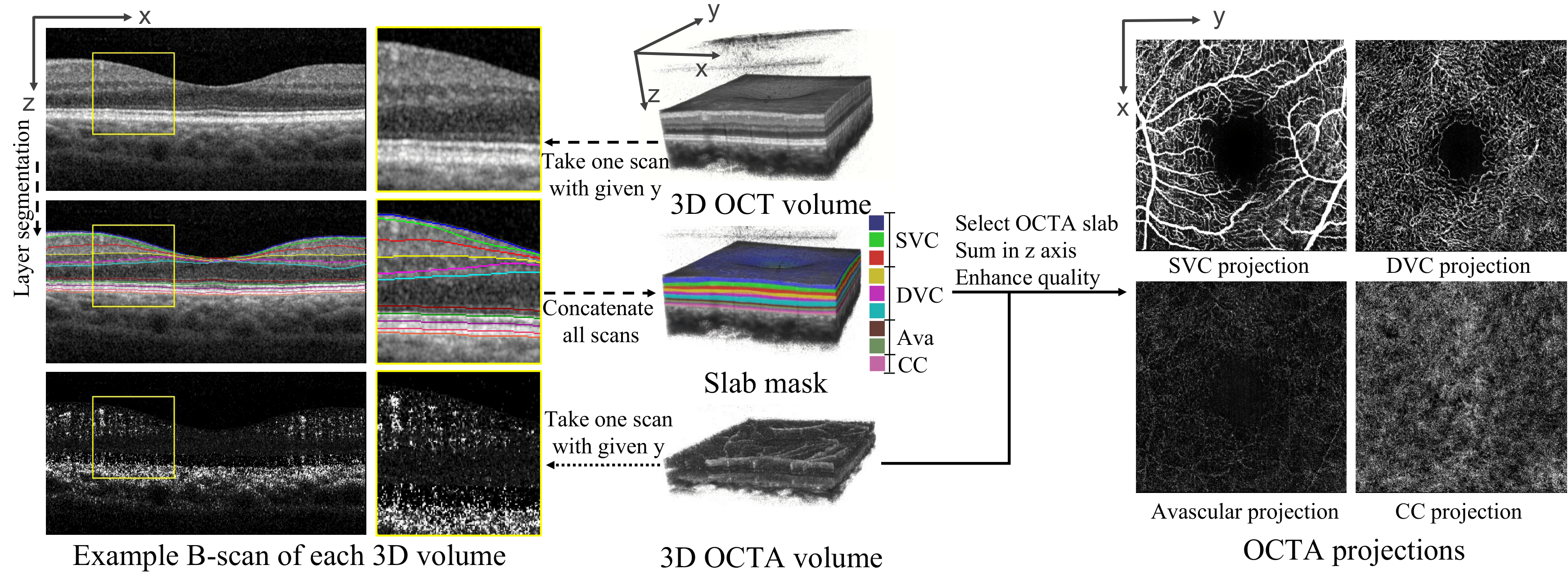
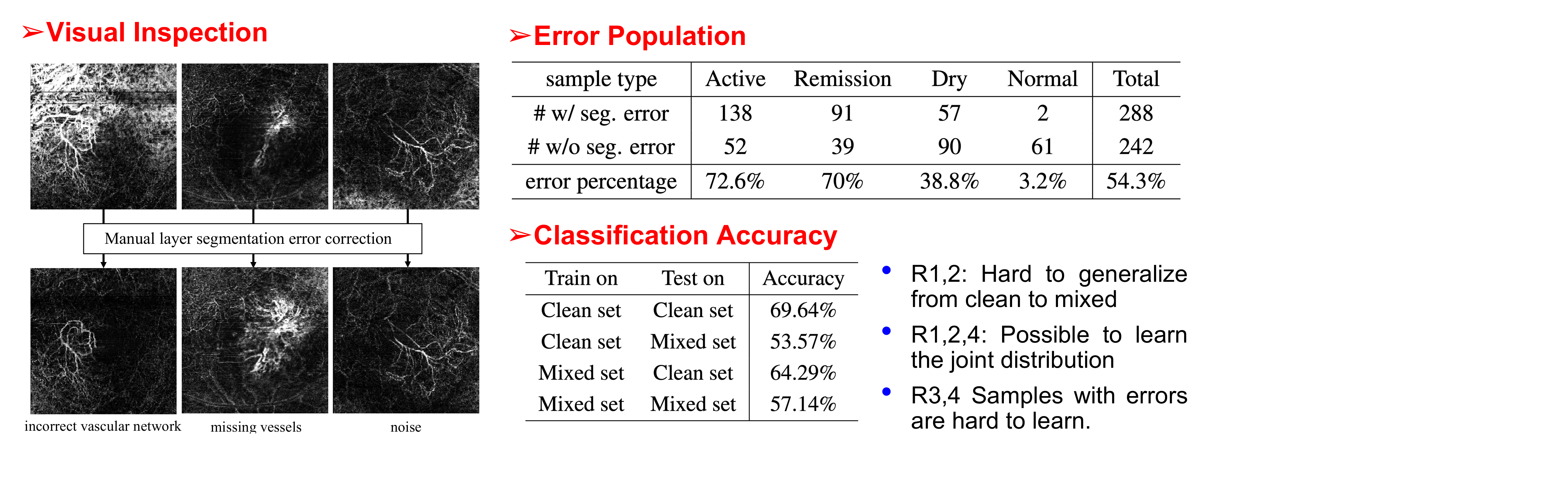
We propose to use 3D raw OCTA volume to avoid the impacts of those errors. To achieve this, we modify a pretrained 2D network to perform volume classification. We also adopt an additional projection supervision to facilitate training of shallow feature extractor.
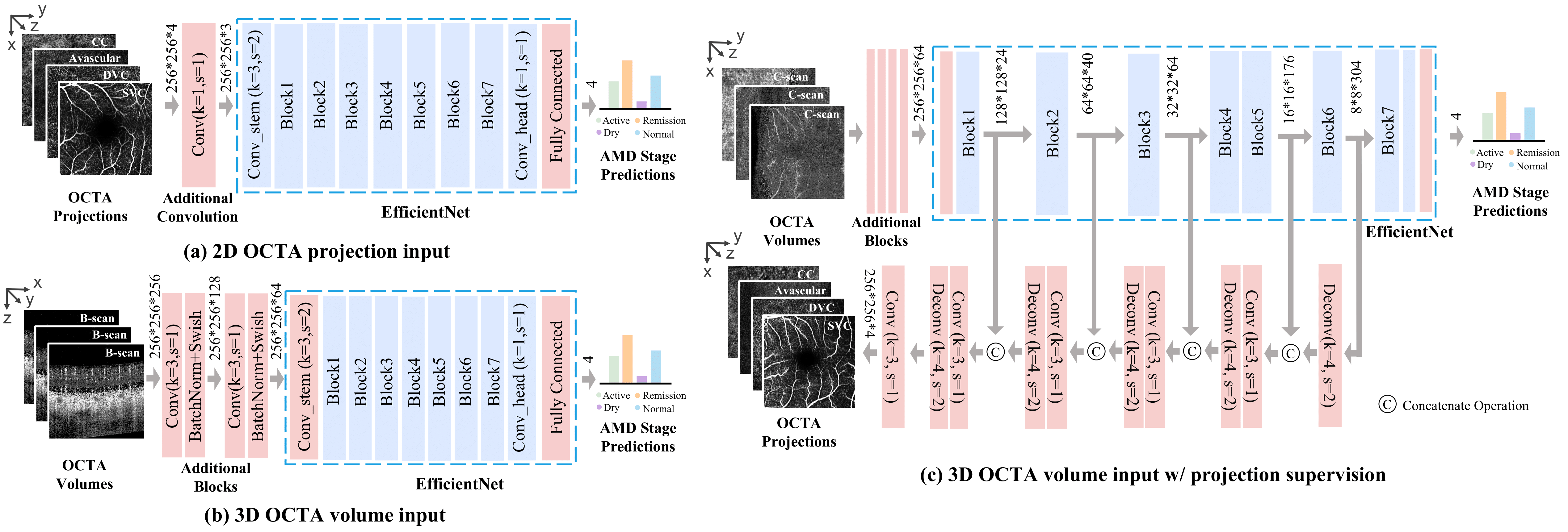
Experimental results show that the proposed classifier can achieve the accuracy of more than 80%, regardless of the presence of layer segmentation errors. These results prove the effectiveness of our methods and suggest that OCTA is a promising modality to distinguish various stages of AMD disease.
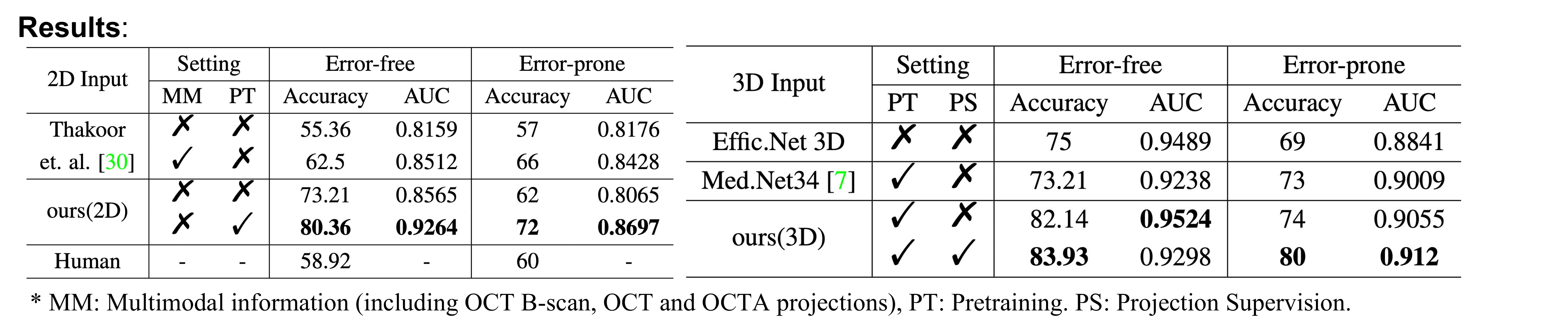
Please also refer to our clinical paper
Citation:
@inproceedings{zhang2023robust,
title={Robust AMD Stage Grading with Exclusively OCTA Modality Leveraging 3D Volume},
author={Zhang, Haochen and Heinke, Anna and Galang, Carlo Miguel B and Deussen, Daniel N and Wen, Bo and Bartsch, Dirk-Uwe G and Freeman, William R and Nguyen, Truong Q and An, Cheolhong},
booktitle={IEEE/CVF International Conference on Computer Vision (ICCV) Workshop},
pages={2411--2420},
year={2023}
}
@article{heinke2022artificial,
title={Artificial intelligence for OCTA-based disease activity prediction in age-related macular degeneration.},
author={Heinke, Anna and Zhang, Haochen and Deussen, Daniel and Galang, Carlo Miguel B and Warter, Alexandra and Kalaw, Fritz Gerald Paguiligan and Bartsch, Dirk-Uwe G and Cheng, Lingyun and An, Cheolhong and Nguyen, Truong and others},
journal={RETINA},
pages={10--1097},
year={2022}
}