Unsupervised Real-World Super-Resolution: A Domain Adaptation Perspective
Published in International Conference on Computer Vision (ICCV), 2021
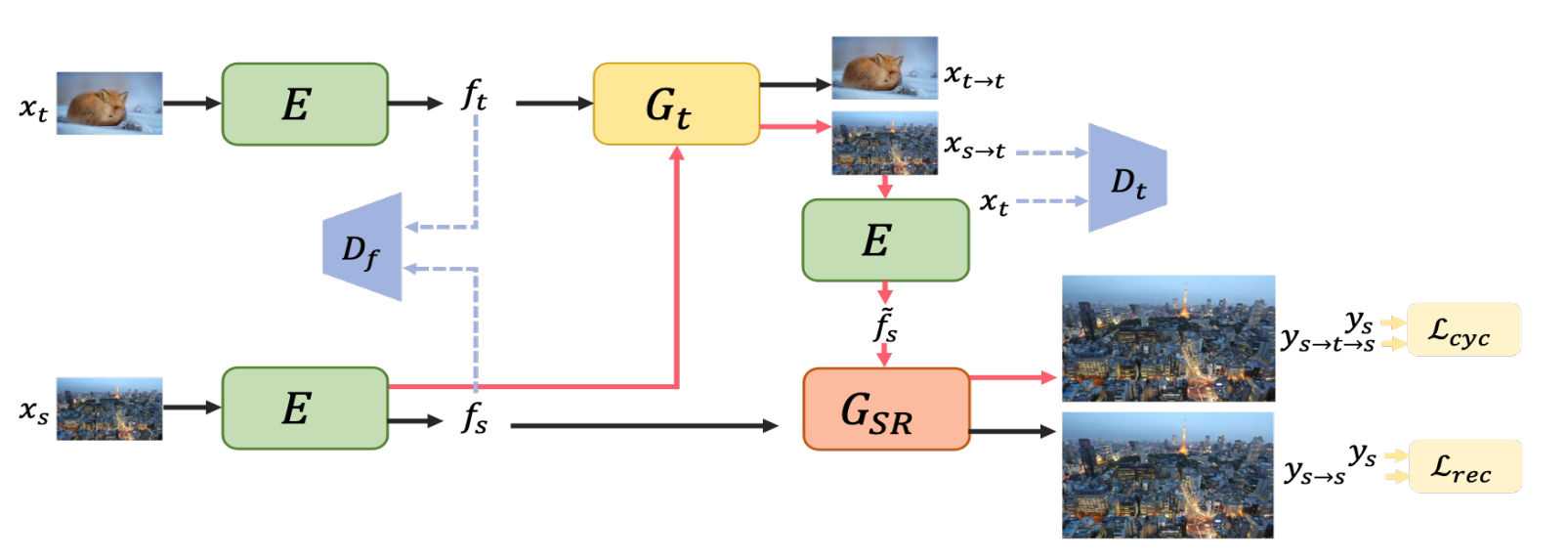
In this paper, we consider the real-world SR problem from the traditional domain adaptation perspective, where the given LR images can be regard as the inputs in target domain and the unpaired HR images can be seen as label in source domain. We propose a novel unpaired SR training framework based on feature distribution alignment, with which we can obtain degradation indistinguishable feature maps and then map them to HR images. In order to generate better SR images for target LR domain, we introduce several regularization losses to force the aligned feature to locate around the target domain, which help to achieve better aligning performance as well as preserve image details for the downstream SR task.
Citation:
@inproceedings{wang2021unsupervised,
title={Unsupervised real-world super-resolution: A domain adaptation perspective},
author={Wang, Wei and Zhang, Haochen and Yuan, Zehuan and Wang, Changhu},
booktitle={IEEE/CVF International Conference on Computer Vision (ICCV)},
pages={4318--4327},
year={2021}
}