On the Classification-Distortion-Perception Tradeoff
Published in Neural Information Processing Systems (NeurIPS), 2019
Distortion corresponds to signal fidelity and perception corresponds to perceptual naturalness, both of which are important metrics in practice. Besides, there is another dimension worthy of consideration–the semantic quality of the restored signal, i.e. the utility of the signal for recognition purpose. In this paper, we analyze the relationship among distortion, perception and semantic quality (classification) in the image restoration tasks. In particular, we consider the classification error rate achieved on the restored signal using a predefined classifier as a representative metric for semantic quality.
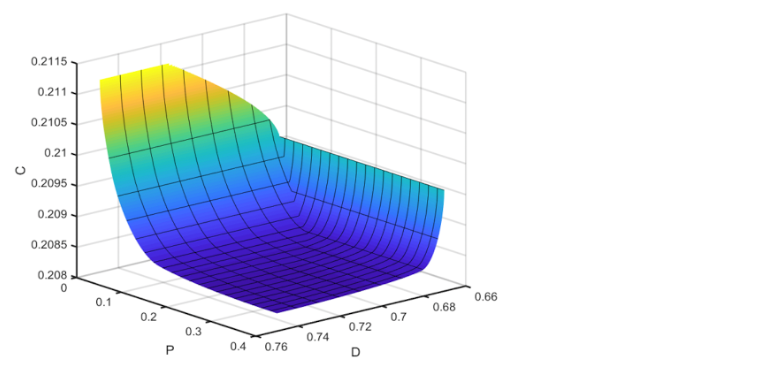
We rigorously prove the existence of the CDP tradeoff, i.e. the distortion, perceptual difference, and classification error rate cannot be made all minimal simultaneously. We also provide both simulation and experimental results to showcase the CDP tradeoff. Our findings can be useful especially for computer vision research where some low-level vision tasks (signal restoration) serve for high-level vision tasks (visual understanding).
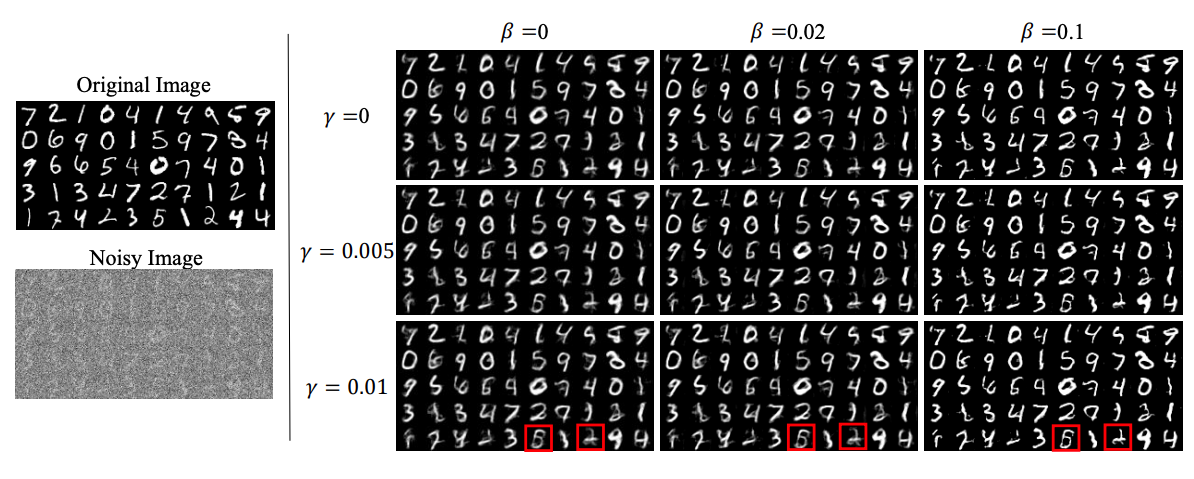
Citation:
@article{liu2019classification,
title={On the classification-distortion-perception tradeoff},
author={Liu, Dong and Zhang, Haochen and Xiong, Zhiwei},
journal={Advances in Neural Information Processing Systems (NeurIPS)},
volume={32},
pages={1--10},
year={2019}
}